Getting conclusive results from A/B tests
UpdatedTesting messages sent to your user base is a fantastic way to learn about your consumers and continue to improve your messaging strategies. But how can you be sure that your tests are actually telling you what you think they’re telling you? When conducting tests, you should aim to maximize the level of confidence you have in the results of the test. This is where statistical significance comes in.
For instance, how do you know that your most engaged users didn’t happen to end up getting the same variation by random chance? Calculating statistical significance is a way of determining how confident you can be that the results of a test are because one variation is more effective than the other.
Let’s take an example: If I want to test whether one email subject line causes more opens than another, and variation A has a 60% open rate and variation B has a 40% open rate, then variation A is the more successful subject line, right? Not necessarily. Understanding a result requires looking at how many people have received each variation and how many performed the desired action.
Imagine that I only sent each variation to 5 people and 3 of the 5 opened variation A, while 2 of the 5 opened variation B. Is a difference of only 1 out of 5 enough to indicate that the opens were actually because of the subject line and not just due to chance? Definitely not.
Now imagine that I sent each variation to 5,000 people. This means that 3,000 opened variation A and 2,000 opened variation B. I’m starting to build a bit more confidence in the fact that the subject line of variation A is the reason that 1,000 more people opened A than B. It’s intuitively clear that the larger numbers provide more confidence, but how confident? 50% chance it wasn’t just random? 90% certain A is better than B?
In an ideal testing world, testers typically aim for 95% confidence in their tests to declare it valid. The more people interact with your messages over time, the higher your confidence level can be. In a marketing world where your primary goal is to get a message out to your users in a timely fashion, you can also choose to accept a lower confidence level in order to make a decision faster. The question you should ask yourself is, “if I aim for an 80% level of confidence, can I really tolerate a 1 in 5 chance that my test is misleading me?” If nothing else, statistical significance can help your team to determine which tests are worthwhile, and which can be skipped because you cannot get a high enough confidence level in the result.
Calculating Statistical Significance
When testing a newsletter with Customer.io, all of the information you need to calculate the confidence level of your test results is provided on the Test tab once the test has started (if you’re testing campaign messages, see Understanding your A/B test results). There are a number of statistical significance calculators that you can use with the information provided. We’ve chosen to use Neil Patel’s calculator for the following example. Note: If you have more than 4 variations in your test, here is one that accommodates up to 10 variations.
If you are testing different subject lines on your emails, it is most likely that success will be defined as the email with the highest open rate. To determine if the difference in the open rates is not by chance, go to the Test tab for the newsletter you are testing and open the statistical calculator of your choice. Enter your test result numbers into the calculator following the guide below:
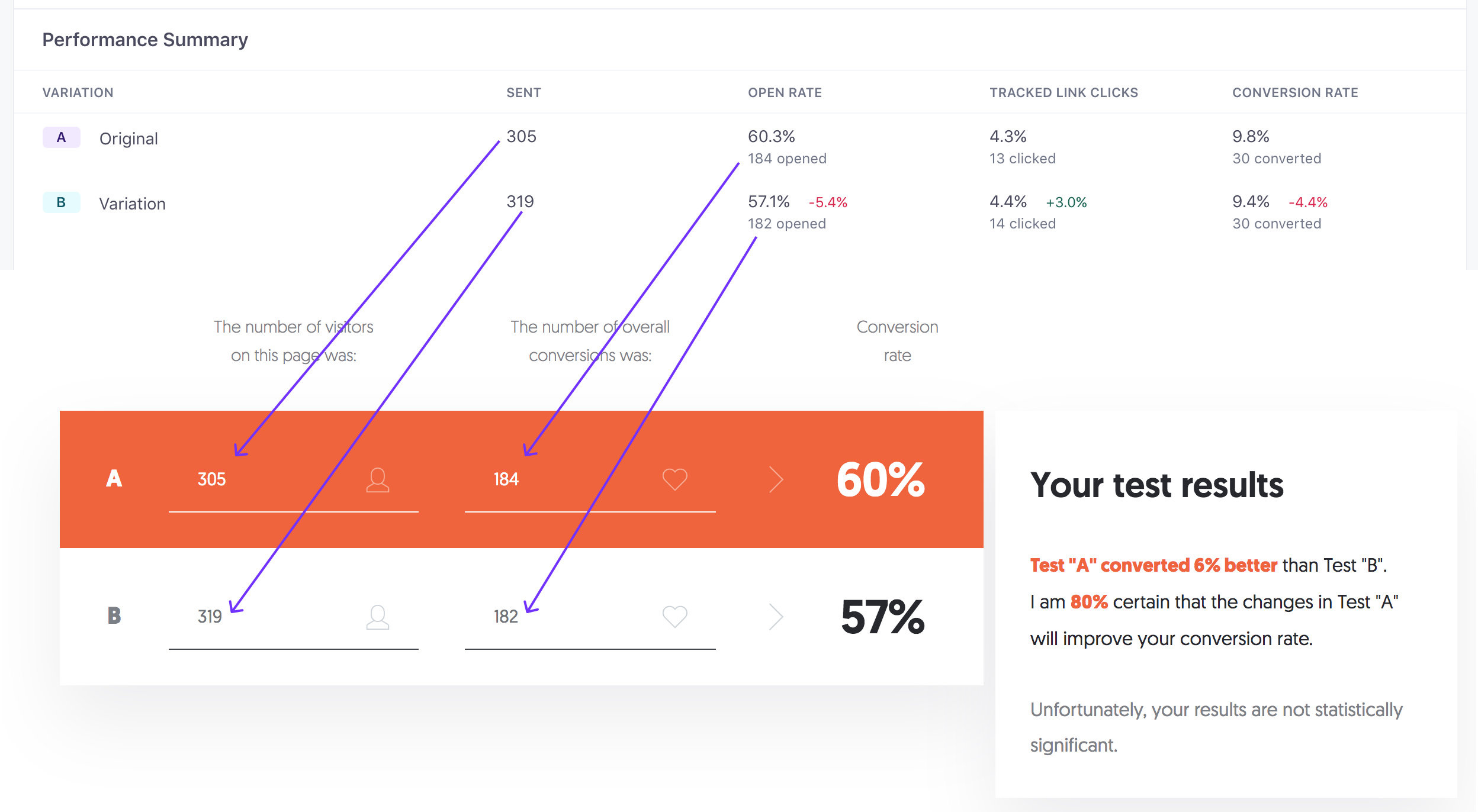
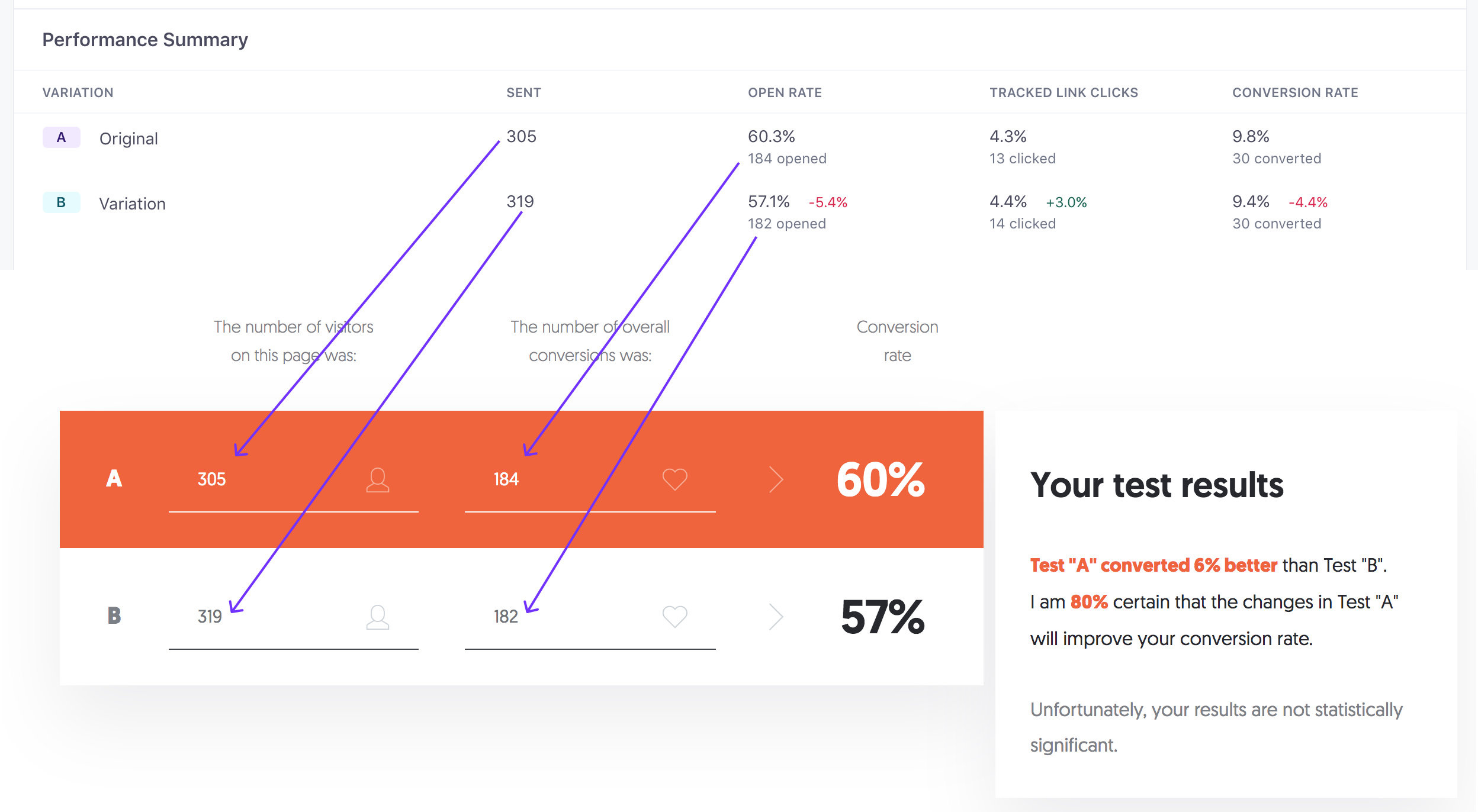
What does the result mean?
Each calculator will have a slightly different way of communicating results, but this calculator uses the phrasing, “I am 90% certain that the changes in Test “A” will improve your conversion rate.” This mean there is a 10% chance that the higher open rates in variation A were just random chance and not a pattern.
How can I increase the likelihood of statistical significance?
We all love being able to declare a winner of a test with high confidence. So how can you increase the number of times your tests are statistically significant? The math that determines significance is driven by 2 primary factors: sample size and test duration.
Sample size
The larger the test’s sample size, the quicker it will achieve the number of email actions required to meet your desired level of confidence. In our world of marketing messages, the quicker we can achieve valid results, the better. If we don’t have large sample sizes, the tests will likely need more time to achieve statistical significance (and if the sample sizes are small enough, they may never get there).
A rule of thumb is a sample size of at least 500 recipients per variation, but it’s worth taking the time to calculate sample sizes specific to your needs and confidence levels using a sample size calculator.
Test duration
Although it is not used in the statistical significance calculation, the duration of a test impacts confidence in the results because the more time users have to act on the email (open it, click it, or convert), the more information you have for calculating the results. When planning how long the test should run, first consider the likelihood that the action will be taken at all. Opening emails is the easiest of the 3 metrics for a user to complete and thus you can expect a greater number of people to do it faster. As you can imagine, the amount of time it takes to gain confidence in the results of a test measuring open rate is much lower than for one measuring conversions.
Armed with at least a basic knowledge of statistical significance and what it means for A/B Testing newsletters, you should be able to increase the productivity of your messaging. Though the concepts and calculations might seem intimidating at a glance, remembering that larger sample sizes and attention to test duration will increase the effectiveness of your tests is a great place to start.